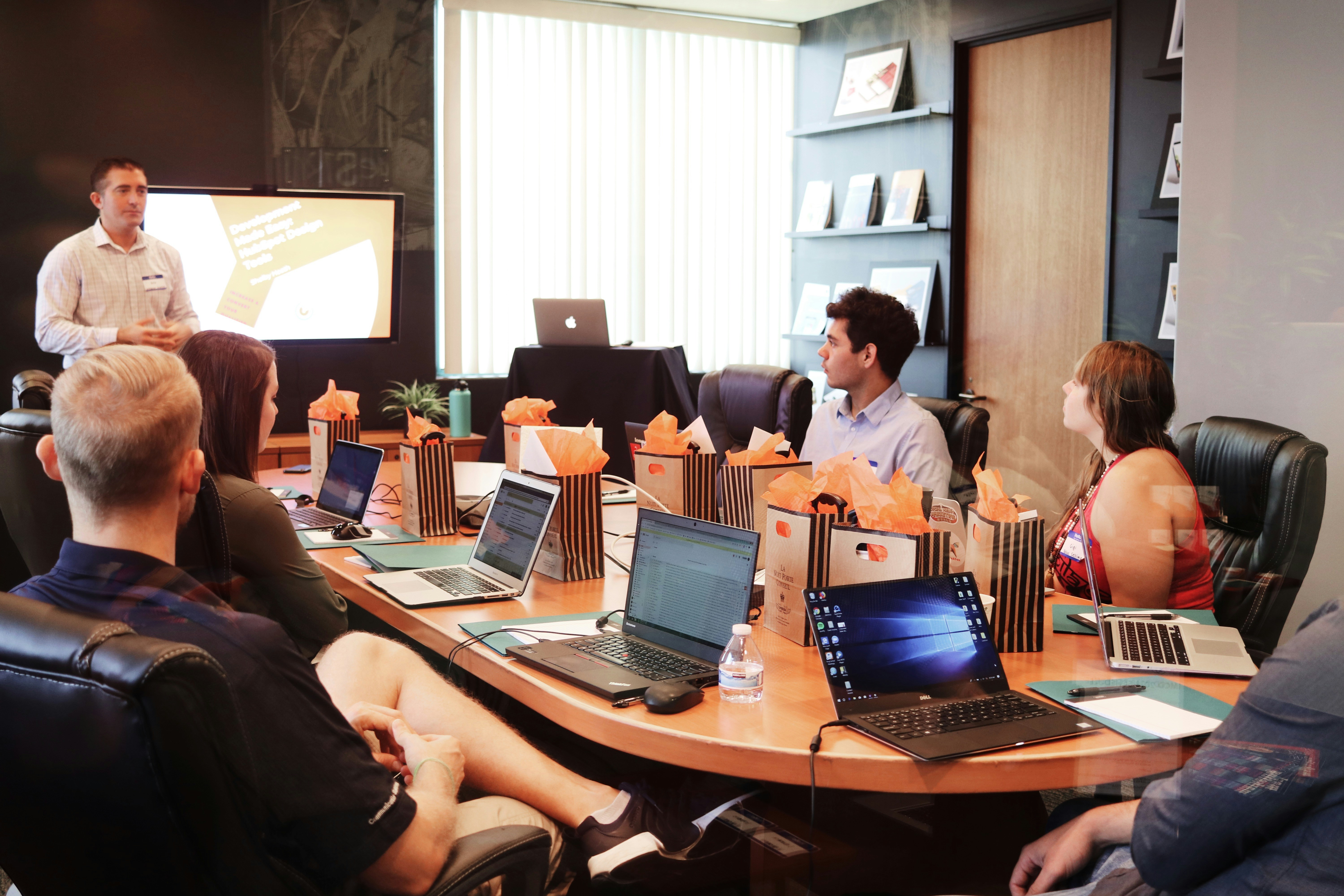
Data Science Jobs for Non‑Technical Professionals: Where Do You Fit In?
Beyond Jupyter Notebooks Ask most people what a data‑science career looks like and they’ll picture Python wizards optimising XGBoost hyper‑parameters. The truth? Britain’s data‑driven firms need storytellers, strategists, ethicists and project leaders every bit as much as they need statisticians. The Open Data Institute’s UK Data Skills Gap 2024 places demand for non‑technical data talent at 42 % of all data‑science vacancies—roles focused on turning model outputs into business value and trustworthy decisions. This guide highlights the fastest‑growing non‑coding roles, the transferable skills many professionals already have, and a 90‑day action plan to land a data‑science job—no pandas required.