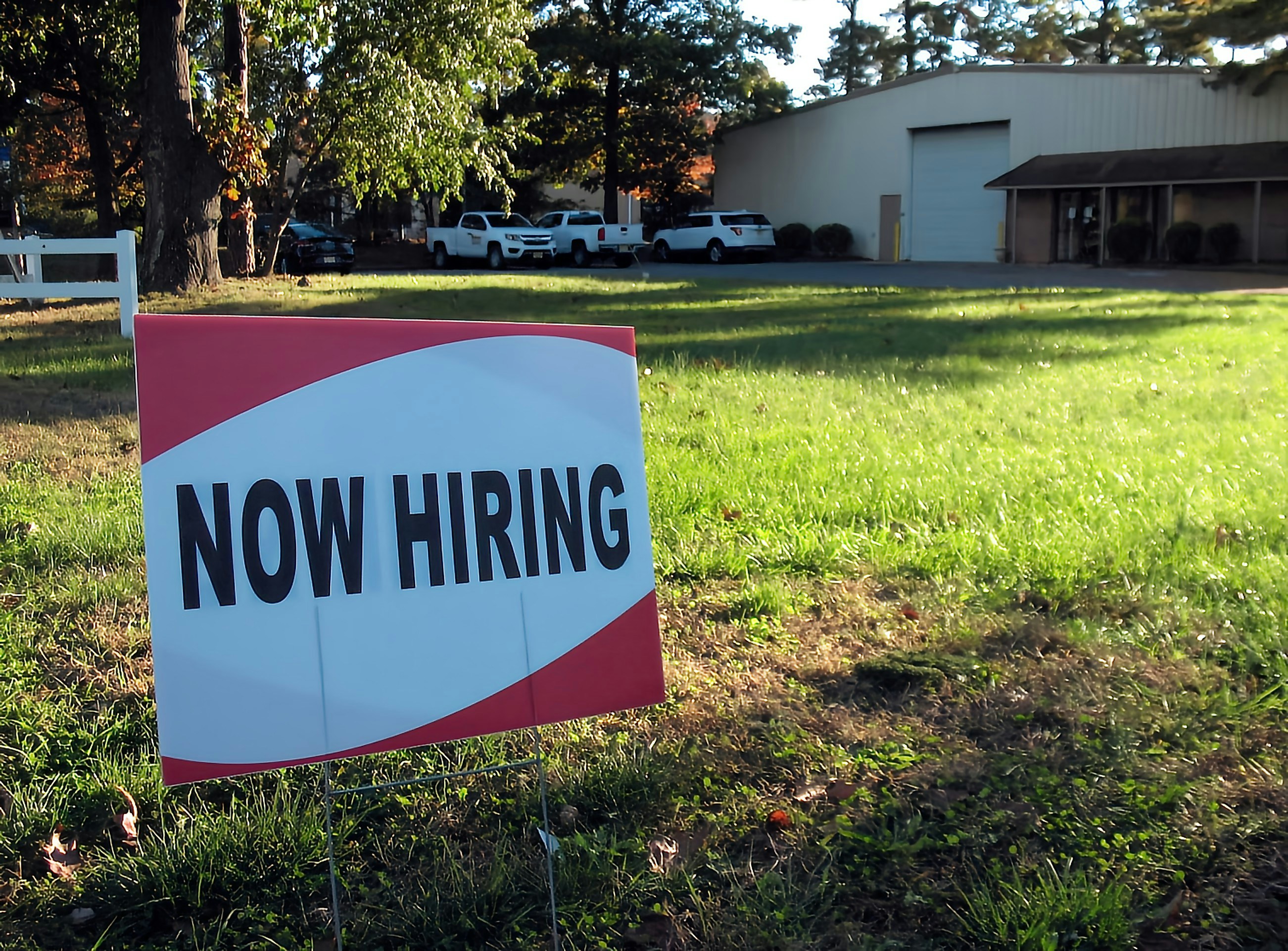
Contract vs Permanent Data Science Jobs: Which Pays Better in 2025?
Data science sits at the intersection of statistics, machine learning, and domain expertise, driving crucial business decisions in almost every sector. As UK organisations leverage AI for predictive analytics, customer insights, and automation, data scientists have become some of the most in-demand professionals in the tech job market. By 2025, data scientists with expertise in deep learning, natural language processing (NLP), and MLOps are commanding top-tier compensation packages. However, deciding whether to become a day‑rate contractor, a fixed-term contract (FTC) employee, or a permanent member of an organisation can be challenging. Each path offers a unique blend of earning potential, career progression, and work–life balance. This guide will walk you through the UK data science job market in 2025, examine the differences between these three employment models, present sample take‑home pay scenarios, and offer strategic considerations to help you determine the best fit for your career.