Related Jobs
View all jobsPrincipal Software Engineer
Machine Learning Engineer (NLP)
Prompt Engineer
Senior Data Engineer (software)
Lead Machine Learning Engineer
Get the latest insights and jobs direct. Sign up for our newsletter.
Industry Insights
Discover insightful articles, industry insights, expert tips, and curated resources.
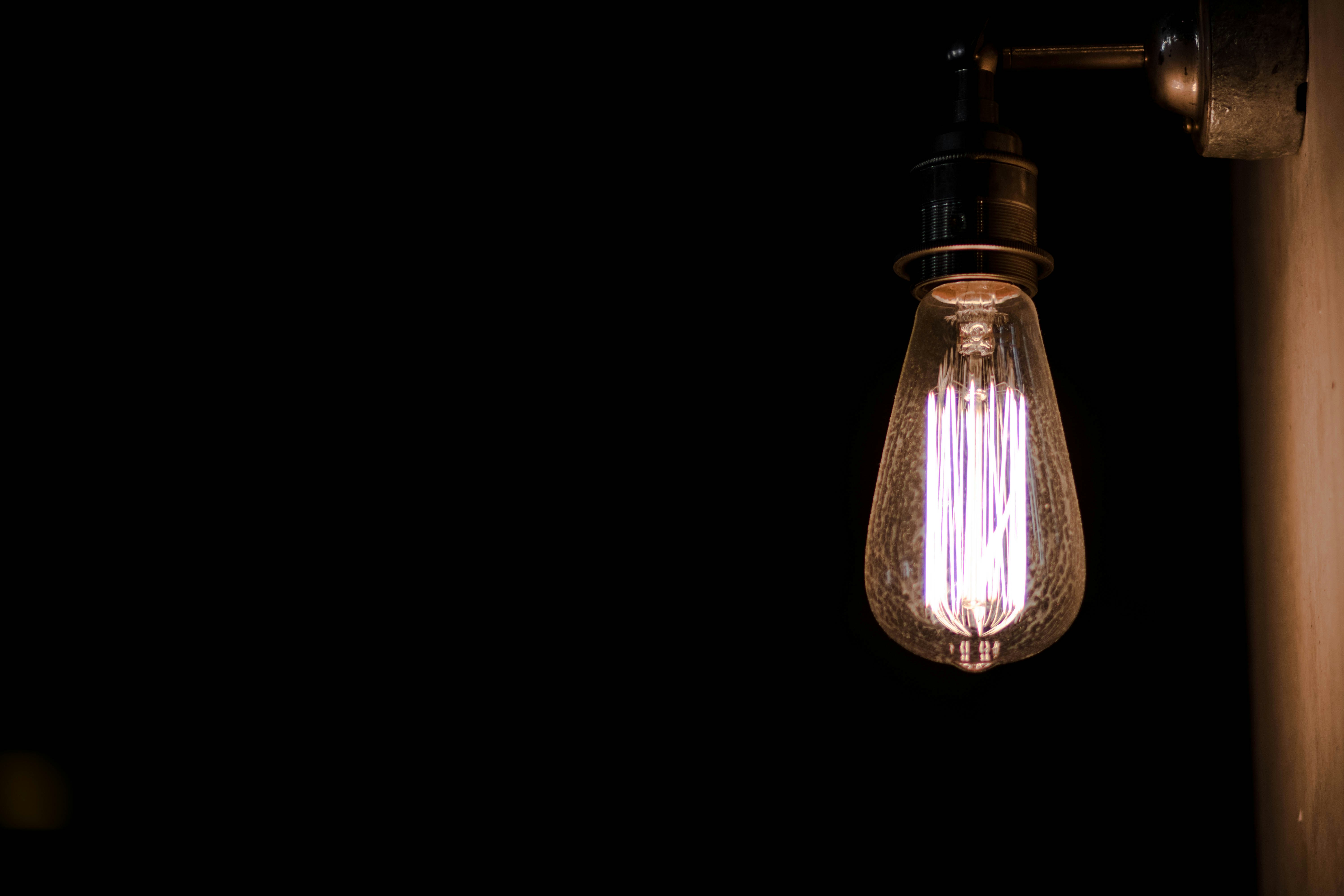
Tips for Staying Inspired: How Data Science Pros Fuel Creativity and Innovation
Data science sits at the dynamic intersection of statistics, computer science, and domain expertise, driving powerful innovations in industries ranging from healthcare to finance, and from retail to robotics. Yet, the daily reality for many data scientists can be a far cry from starry-eyed talk of AI and machine learning transformations. Instead, it often involves endless data wrangling, model tuning, and scrutiny over metrics. Maintaining a sense of creativity in this environment can be an uphill battle. So, how do successful data scientists continue to dream big and innovate, even when dealing with the nitty-gritty of data pipelines, debugging code, or explaining results to stakeholders? Below, we outline ten practical strategies to help data analysts, machine learning engineers, and research scientists stay inspired and push their ideas further. Whether you’re just starting out or looking to reinvigorate a long-standing career, these pointers can help you find fresh sparks of motivation.
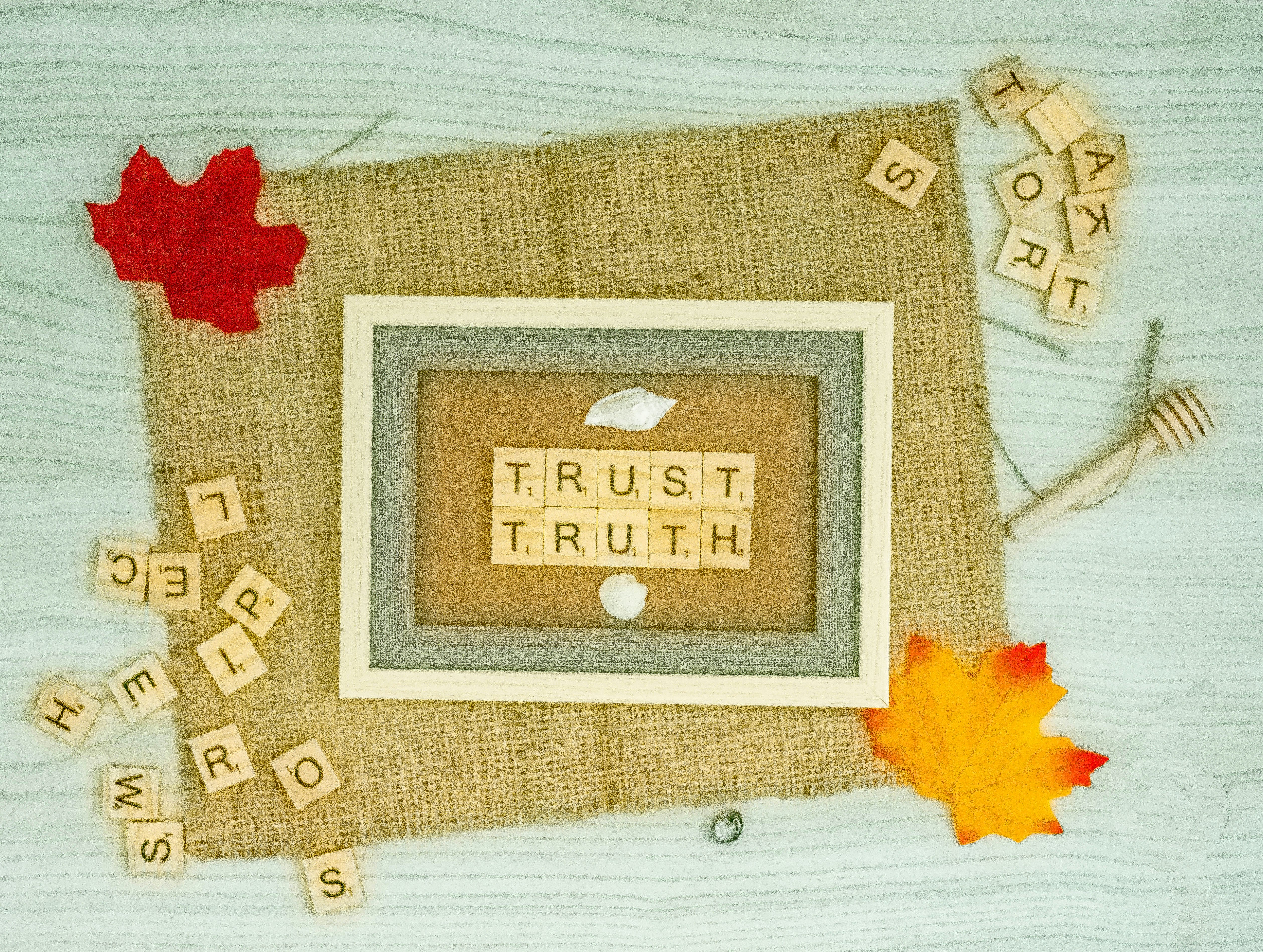
Top 10 Data Science Career Myths Debunked: Key Facts for Aspiring Professionals
Data science has become one of the most sought-after fields in the tech world, promising attractive salaries, cutting-edge projects, and the opportunity to shape decision-making in virtually every industry. From e-commerce recommendation engines to AI-powered medical diagnostics, data scientists are the force behind innovations that drive productivity and improve people’s lives. Yet, despite the demand and glamour often associated with this discipline, data science is also shrouded in misconceptions. Some believe you need a PhD in mathematics or statistics; others assume data science is exclusively about machine learning or coding. At DataScience-Jobs.co.uk, we’ve encountered a wide array of myths that can discourage talented individuals or mislead those exploring a data science career. This article aims to bust the top 10 data science career myths—providing clarity on what data scientists actually do and illuminating the true diversity and inclusiveness of this exciting field. Whether you’re a recent graduate, a professional looking to pivot, or simply curious about data science, read on to discover the reality behind the myths.
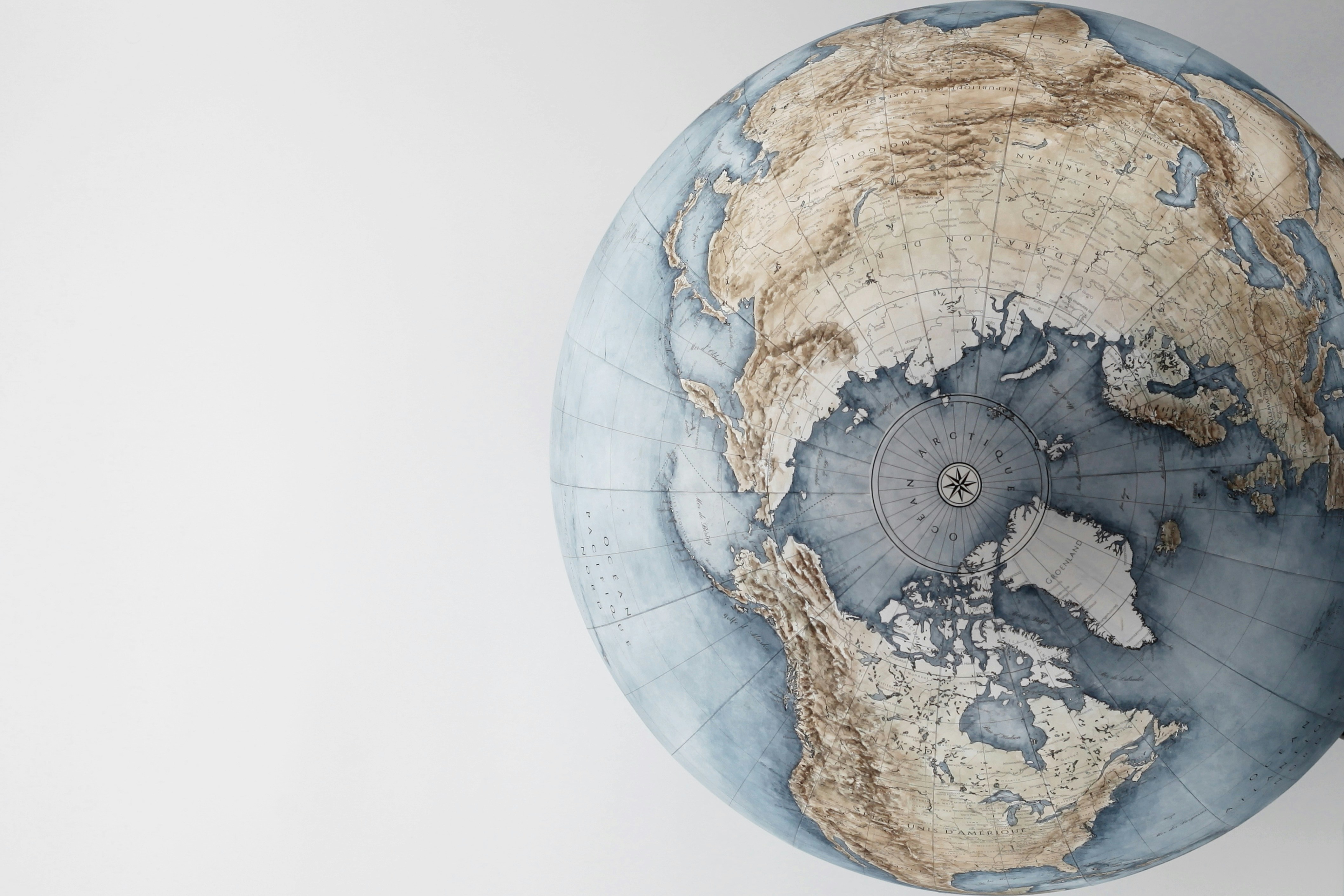
Global vs. Local: Comparing the UK Data Science Job Market to International Landscapes
How to evaluate salaries, opportunities, and work culture in data science across the UK, the US, Europe, and Asia Data science has proven to be more than a passing trend; it is now a foundational pillar of modern decision-making in virtually every industry—from healthcare and finance to retail and entertainment. As the volume of data grows exponentially, organisations urgently need professionals who can transform raw information into actionable insights. This high demand has sparked a wave of new opportunities for data scientists worldwide. In this article, we’ll compare the UK data science job market to those in the United States, Europe, and Asia. We’ll explore hiring trends, salary benchmarks, and cultural nuances to help you decide whether to focus your career locally or consider opportunities overseas or in fully remote roles. Whether you’re a fresh graduate looking for your first data science position, an experienced data professional pivoting from analytics, or a software engineer eager to break into machine learning, understanding the global data science landscape can be a game-changer. By the end of this overview, you’ll be better equipped to navigate the expanding world of data science—knowing which skills and certifications matter most, how salaries differ between regions, and what to expect from distinct work cultures. Let’s dive in.